Evaluating the uncertainties of nature information
All data collected from nature contains uncertainty. Despite this, measurements and monitoring that produce natural data provide valuable insights into the state of various biological groups, ecosystems, or habitat types. In decision-making based on natural data, it is important to consider the uncertainty of the information and its suitability for each specific purpose.
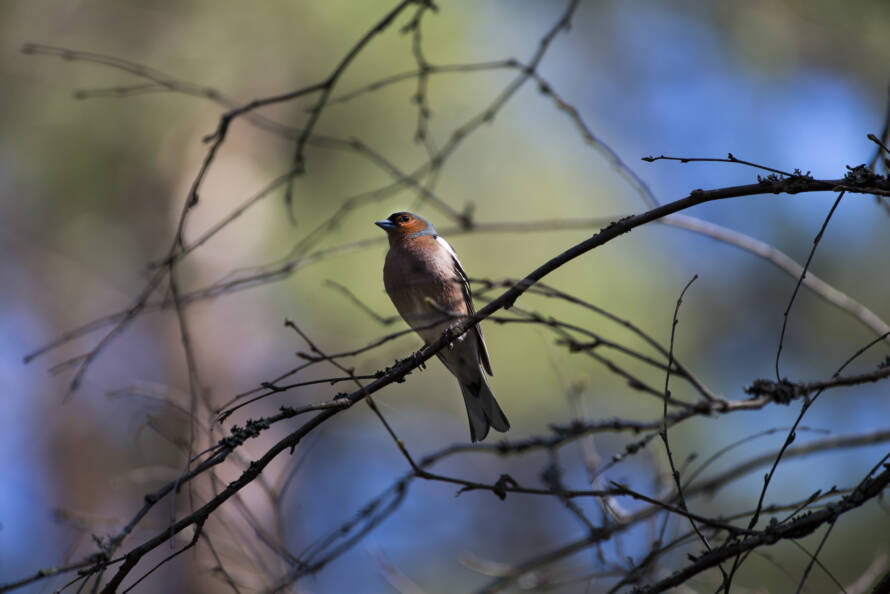
How can data reliability be taken into account in the use of nature data?
The sources and occurrences of uncertainty in nature information vary depending on the source and type of he information. In practice, uncertainty usually means that the thing being measured is not known exactly, but the information is, on average, correct. When conclusions and recommendations are made on the basis of measured nature data, uncertainty can be assessed and accounted for by various methods. The most appropriate methods for different situations depend on the characteristics of the data. Systematic error is of a different nature from the uncertainty described above. In that case, the data is systematically skewed and therefore difficult to use to support conclusions. Therefore, the assessment and reporting of various uncertainties is an essential part of producing high quality nature data.
The user of nature information must take the following things into account:
- The uncertainty of the measured data can be expressed as a numerical value or as qualitative data.
- Observations collected by random sampling must be based on a well-planned sample, otherwise there is a risk of systematic error.
- When using map-based observations, it must be taken into account whether there is information only about the places where the measured object has been observed, or whether there is also information about places where the object has not been observed.
- Measured information is usually obtained only at certain times and from certain places – however, the lack of temporal and spatial information can be fixed with the help of modeling.
- The accuracy of the modeled nature data is affected by the uncertainty related to the modeling methods.
Assessing uncertainty
In the natural sciences, the reliability of quantitative data is assessed by defining a range for the uncertainty of the results. Uncertainty can often be reduced by increasing the number of measurement points, by performing quality assurance of measurements or by improving modeling. Still, it is usually not even possible to reach full certainty or accuracy. In some cases, the amount of uncertainty can also be expressed as a qualitative estimate, if an exact numerical value cannot be produced.
Example 1. – Confidence interval
A 95 percent confidence interval is reported for several indicators based on extensive monitoring data presented in the Luonnontila web service. This means that the generalizable value of the indicator is with a 95 percent probability within that confidence interval. For example, the chemical oxygen consumption of humus-rich lakes(you are switching to another service) was between 12.6 and 10.1 mg/l in 2023 with a 95 percent probability. The average annual speed and direction of development for the indicators is also evaluated, and for this a 90 percent confidence interval, which is used to classify the indicator’s development.(you are switching to another service)
The sample affects the reliability of the observations
Monitoring of nature observations can be done systematically, based on well-planned sampling and a sufficiently large sample. The findings from such monitoring can be considered comprehensive and representative.
The sample, i.e. the selection of observation units, can be made by means of random selection in such a way that the observation points comprehensively reflect the characteristics of the distribution areas of the population to be measured. If some type of observation unit is favored in the sample or excluded from the sample, the observations may be biased, which reduces the reliability of the results. There should also be enough observation points in relation to the occurrences of the observed species. With a small sample size, the uncertainty of the conclusions drawn from the observations increases, while increasing the sample size decreases it.
Another frequently used method of collecting nature observations is systematic sampling, where regionally comprehensive samples are collected of the nature feature to be observed by systematically placing survey points or lines of a certain size or length, and always at a certain distance from each other.
Example 2. – Line transect sampling
The monitoring of changes in breeding birds is mainly based on line transect method, which is carried out as a systematic sampling. Usually, rectangular six-kilometer-long permanent lines are placed about every 25 kilometers across Finland, and there are a total of 566 of these lines. The lines are repeated every 2-3 years so that 200-300 lines are counted each year. Based on the lines, it is possible to calculate the annual breeding season density for each species and population change in the desired time interval. However, population change indices can only be calculated for species for which sufficient observational data is accumulated in the calculations. For species with few numbers, such as many birds of prey, the data in these calculations is too small, so these species have their own species-specific monitoring.
Example 3. – Diversity of species groups
The diversity of some species groups is so extensive that getting an overall picture of them is a considerable challenge in species monitoring. The monitoring and observation of butterflies is organized in an exemplary manner in Finland, and it includes point- or area-specific occurrence data collected through citizen observations, line transect data collected through systematic guidelines, and species data collected from certain survey plots. All in all, this is valuable species monitoring data. Along with the butterflies, however, there is a wide range of micro-butterfly species that are challenging to identify and often harder to spot than the butterfly species. Due to the smaller number of hobbyists specializing in micro-butterflies, their monitoring data contain regional gaps of varying sizes, and the knowledge of their population changes is less certain than that of larger butterflies.
Recording observations made in species monitoring and also recording the absence of observations affect the coverage of information derived from measurements. Systematic monitoring provides information on whether a species is present at sampling points or study areas or whether it is missing. In this way, valuable information can also be obtained on sites where certain species are missing. In many surveys based on citizen observations, information is obtained only about the species that have been observed. In this case, no information is obtained from the areas where the species has not been observed, which must be considered when interpreting the collected data. If it is not taken into account, there is a risk of skewing the calculations and conclusions made from the monitoring data, which can lead to systematic over- or underestimations.
Example 4. – Modeling
When monitoring data containing only positive presence observations of species is used as a starting point for modeling, this limitation must be taken into account when applying the modeling method. One method that can use only positive species sightings is Maxent. When using this method, however, another uncertainty factor must be taken into account, i.e. the spatial biases of occurrence observations, the correction of which requires the use of variables that correct the spatial weighting of occurrences. One example of this is the distribution models made in Finland for six forest indicator bird species. They are based on observations of the nests of these species, from which chicks had been ringed, as well as tree structure and land cover data. In these models, the spatial biases of the nest data were corrected using a correction factor calculated based on the species’ nest locations.
The abundance of the species and basic information about the location of the species affect the number of observations. If there is not enough information about the location, or the species is really rare, only a few observations can be made. This increases the uncertainty of the results. However, some rare groups of organisms or habitat types and the location of their occurrence are well known, so even with a small sample one can get accurate information about the present situation. On the other hand, for less well-known, rare groups of organisms, a small sample can mean great uncertainty.
Temporal and spatial coverage of observations
Observations typically only cover part of the studied area. In addition, nature observations normally do not form a continuous series in time but are accumulated from individual points in time. Many species and groups of organisms are monitored on the basis of samples such as this, which are limited in location and time. However, statistical indicators can be calculated from these samples, such as averages of the number of observations in some area, and the development of these indicators over time can be monitored. In addition to the average value, the uncertainty of the sample can be calculated. The uncertainty of the mean usually decreases as the sample size increases.
If there are only a few observations, it can be difficult to draw conclusions because of the high uncertainty. In this case, for example, even a large change between the averages of observations made at consecutive moments can be small in relation to the uncertainty. Then it cannot be said with certainty whether a change has really taken place.
With the help of individual, spatially random observations of groups of organisms or ecosystems, it is possible in certain cases to produce estimates of their condition even for large areas. If it is possible to mathematically model the ecological features of the occurrence of a certain group of organisms, its population dynamics and its interaction relationships, it is also possible to obtain comprehensive estimates of its changes over time. Regarding modeling results, it is also important to consider their uncertainty.
Example 5. – Remote sensing
There are great hopes that remote sensing could improve the coverage of nature information. In particular, using spectrum and laser scanning data collected from the air, i.e. by airplane or drones, it is possible to determine structural features of the habitat important to many species. Such variables include the height of vegetation, the size of trees and tree species. In a study published in 2023 by the Finnish Environment Institute and the University of Eastern Finland, it was found that models using such remote sensing variables that describe a wider habitat, predicted the lichen species growing on the trunk of the wood ash better than models based on the characteristics measured in the terrain of individual aspen trees. With remote sensing, it is possible to cost-effectively map potentially diverse areas in terms of species, but confirmed terrain observations are still necessary to verify the species and as teaching material for the models.
Uncertainties of field observations and measurements
Uncertainty in measurements comes from, for example, the inaccuracy of the measurement methods, the characteristics of the object to be measured, as well as the rounding errors of the results. In addition, field measurements in particular may include uncertainty as to whether the organisms of the entire observation area have been detected. The number of observations may vary depending on weather conditions, time of day and other environmental factors.
For some species, the measurement results can be very precise (for example, the number of large, highly visible and rare mammals), while assessment of the number or distribution of small or hiding targets or targets with varying distibution (microbes, small-sized species) is already uncertain in itself. The identification of habitat types in the field can be uncertain, especially in small-sized occurrence areas or in places with strong, multidimensional ecological variation; the same point can get different definitions from different nature surveyors.
Example 6. – Study areas
For long-term monitoring of vegetation, fixed test areas/squares are often established. Monitoring is done annually at the same penological time, because a difference of even a couple of weeks can be seen as the absence of some short-lived species. There is also a natural variation between years in the presence of plants. Evaluating coverage is always subjective and there are differences between surveyors.
New species detection methods based on DNA sequencing or automatic image or sound recognition do not depend on the observer’s knowledge of the species and are therefore more objective and repeatable than traditional observation. However, even such observations are subject to uncertainty. The most important sources of uncertainty in these so-called machine observation methods have shortcomings in the coverage of reference material such as DNA sequence libraries or bird sound material used in teaching an artificial intelligence algorithm. If a rare species is poorly represented in the reference material, its identification is easily left uncertain, especially if there is also significant variation within the species, such as in the case of bird sounds. In the case of DNA methods, the reliability of the observation is also affected by numerous methodological details, from the preservation of the sample to the primers used in DNA duplication. In addition, there can be uncertainty about the origin of DNA detected in an environmental sample such as water or air. Especially in the air and in large rivers, DNA can travel to the sample from considerable distances. Dispersal modeling that takes air and water flows into account can be used to identify possible source areas.
Example 7. – Measurement practices
Uniform measurement practices ensure the quality of species monitoring. For example, bird counts during breeding season are only done in good weather conditions in the early morning, and the aim is to make the counts of the same area at the same phenological time every year. The calculations are also done regionally at a slightly different time, i.e. earlier in June in southern Finland than in northern Finland, due to differences in phenology in different parts of the country. The recommended weather conditions must also be obeyed in line transects of butterflies in order to collect standardized data. Weather that is too cool or cloudy leads to a decrease in the activity of butterflies, and there is a risk that the collected data will be deficient due to weather conditions.
Example 8. – Time
Fixed research areas are typically established for long-term monitoring of vegetation. Estimating the coverage of plant species in small-sized areas is more likely to produce more accurate results than in large-sized areas, but in follow-up studies, when surveyors change, it is important to ensure that the coverage estimates are not biased depending on the person even in the large-sized areas. With large squares, the assessment of coverage can be a considerable challenge, in which case abundance estimates can be made for species by dividing the square into small sub-squares and calculating the species’ frequency of occurrence in them. Another option is to use abundance classes. It is also important to carry out surveys regarding vegetation phenology at the same time, or alternatively several times during the growing season; short-term spring bloomers and early summer species may falsely appear to be in decline if the most recent surveys were made at a later time than the previous ones.
Uncertainty of modeling
In nature data that covers a large area, part of the information is often based on modeling, because the observations themselves are only located in a limited number of observation points. In other respects, the spatial information of the area can be modeled if the observed object is correlated with a variable that can be measured using, for example, remote sensing. The observation data itself can also be the result of modeling, if the observation data is not a variable that can be measured.
Example 9. – Classification model
The information collected by remote sensing can be used together with the information collected in the field to model for example land cover or habitat types. This is so-called classification model, where the goal of the modeling is to find the most probable class for each object on the ground in the research area, which can be a single image pixel or a delimited pattern. Ideally, for each land cover class/nature type, a comprehensive and representative reference material has been collected in the field, which can be divided into two parts (e.g. 70% and 30%) so that one part is used to train the model and the other part is left aside for the assessment of classification uncertainty. When data that the model has not seen before is used to validate the model, not only is a more realistic assessment of the model’s accuracy obtained, but also an assessment of how well the classification model scales to other data and areas.
To evaluate the accuracy of the classification model, the so-called confusion matrix is used, in which the results predicted by the model are compared with actual field observations by category. The accuracy of the classification can be evaluated using several different metrics. The overall accuracy is obtained from the ratio of correctly classified observations to the number of all observations, which, however, alone does not give a true picture, especially if the class distribution is uneven. Class-specific accuracy values can also be derived from the confusion matrix, which provide in-depth information about the classes for which the classification is or is not reliable. These include the producer’s recall and the user’s precision, which also take into account false positive and false negative predictions. In other words, the producer’s recall tells the probability that observations that actually belong to a category are classified as belonging to this category, while the user’s precision tells the probability that the observation belongs to its predicted category.
Nature data, which is based on modeling, contains uncertainties from several different sources. Uncertainty related to the starting material of the modeling, the parameters of the modeling, and the model error appear as the uncertainty of the modeling results.
The uncertainty of future model forecasts may also depend on external factors, such as local weather and land use, about which there is no precise information. Their variation can be described, for example, with scenario models in which assumptions have been made for changes in external factors and their uncertainties.